Pandas
Understanding pandas
errors
pandas
errors can look red, scary, and very long. Fortunately, we don’t need to understand the entire thing! The most important parts of an error message are at the top, which tells you which line of code is causing the issue, and at the bottom, which tells you exactly what the error message is.
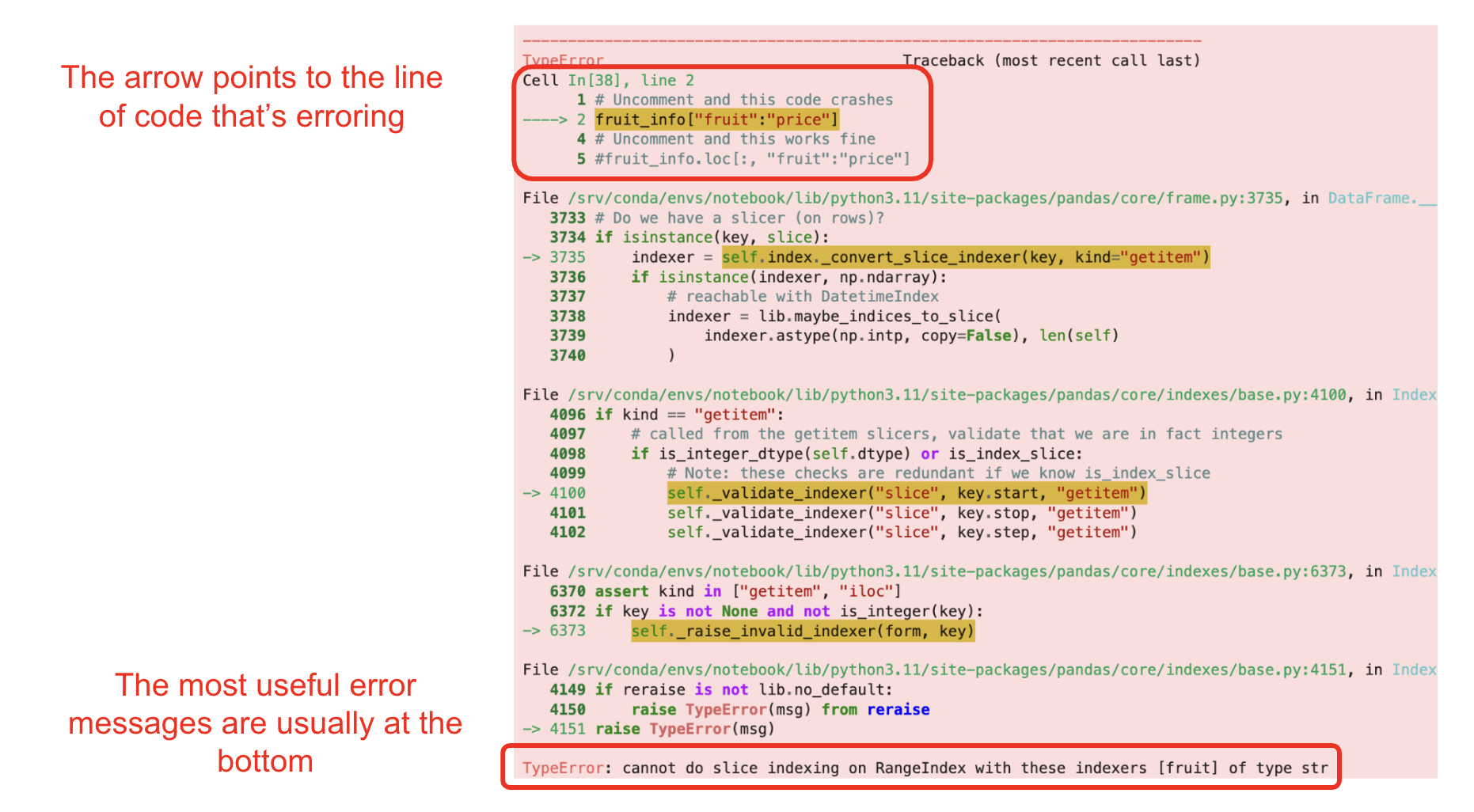
This note is (mostly) structured around the error messages that show up at the bottom.
My code is taking a really long time to run
It is normal for a cell to take a few seconds – sometimes a few minutes – to run. If it’s is taking too long, however, you have several options:
- Try restarting the kernel. Sometimes, Datahub glitches or lags, causing the code to run slower than expected. Restarting the kernel should fix this problem, but if the cell is still taking a while to run, it is likely a problem with your code.
- Scrutinize your code. Am I using too many for loops? Is there a repeated operation that I can substitute with a
pandas
function?
Why is it generally better avoid using loops or list comprehensions when possible?
In one word: performance. NumPy
and pandas
functions are optimized to handle large amounts of data in an efficient manner. Even for simple operations, like the elementwise addition of two arrays, NumPy
arrays are much faster and scale better (feel free to experiment with this yourself using %%time
). This is why we encourage you to vectorize your code (ie. using NumPy
arrays, Series
, or DataFrames
instead of Python lists) and use in-built NumPy
/pandas
functions wherever possible.
KeyErrors
KeyError: 'column_name'
This error usually happens when we have a DataFame
called df
, and we’re trying to do an operation on a column 'column_name'
that does not exist. If you encounter this error, double check that you’re operating on the right column. It might be a good idea to display df
and see what it looks like. You could also call df.columns
to list all the columns in df
.
TypeErrors
TypeError: '___' object is not callable
This often happens when you use a default keyword (like str
, list
, range
, bool
, sum
, or max
) as a variable name, for instance:
sum = 1 + 2 + 3
These errors can be tricky because they don’t error on their own but cause problems when we try to use the name sum
(for example) later on in the notebook.
To fix the issue, identify any such lines of code (Ctrl+F on “sum =
” for example), change your variable names to be something more informative, and restart your notebook.
Python keywords like str
and list
appear in green text, so be on the lookout if any of your variable names appear in green!
TypeError: could not convert string to a float
This error often occurs when we try to do math operations (ie. sum
, average
, min
, max
) on a DataFrame
column or Series
that contains strings instead of numbers (note that we can do math operations with booleans; Python treats True
as 1 and False
as 0).
Double check that the column you’re interested in is a numerical type (int
, float
, or double
). If it looks like a number, but you’re still getting this error, you can use .astype(...)
(documentation) to change the datatype of a DataFrame
or Series
.
TypeError: Could not convert <string> to numeric
Related to the above (but distinct), you may run into this error when performing a numeric aggregation function (like mean
or sum
functions that take integer arguments) after doing a groupby
operation on a DataFrame
with non-numeric columns.
Working with the elections
dataset for example,
elections.groupby('Year').agg('mean')
would error because pandas
cannot compute the mean of the names of presidents. There are three ways to get around this:
- Select only the numeric columns you are interested in before applying the aggregation function. In the above case, both
elections.groupby('Year')['Popular Vote']
orelections['Popular vote'].groupby('Year')
would work. - Setting the
numeric_only
argument toTrue
in the.agg
call, thereby applying the aggregation function only to numeric columns. For example,elections.groupby('Year').agg('mean', numeric_only=True)
. - Passing in a dictionary to
.agg
where you specify the column you are applying a particular aggregation function to. Continuing the same example, this looks likeelections.groupby('Year').agg({'Popular vote' : 'mean')
.
TypeError: 'NoneType' object is not subscriptable
/ AttributeError: 'NoneType' object has no attribute 'shape'
This usually occurs as you assign a None
value to a variable, then try to either index into or access some attribute of that variable. For Python functions like append
and extend
, you do not need to do any variable assignment because they mutate the variable directly and return None
. Assigning None
tends to happen as a result of code like:
some_list = some_list.append(element)
In contrast, an operation like np.append
does not mutate the variable in place and, instead, returns a copy. In these cases, (re)assignment is necessary:
some_array = np.append(some_array, element)
TypeError: 'int'/'float' object is not subscriptable
This occurs when you try and index into an integer or other numeric Python
data type. It can be confusing to debug amidst a muddle of code, but you can use the error message to identify which variable is causing this error. Using type(var_name)
to check the data type of the variable in question can be a good starting point.
IndexErrors
IndexError: invalid index to scalar variable.
This error is similar to the last TypeError
in the previous section. However, it is slightly different in that scalar variables come up in the context of NumPy
data types which have slightly different attributes.
For a concrete example, if you defined
numpy_arr = np.array([1])
and indexed into it twice (numpy_arr[0][0]
), you would get the above error. Unlike a Python integer whose type is int
, type(numpy_arr[0])
returns the NumPy
version of an integer, numpy.int64
. Additionally, you can check the data type by accessing the .dtype
attribute of NumPy
array (numpy_arr.dtype
) or scalar variable (numpy_arr[0].dtype
).
IndexError: index _ is out of bounds for axis _ with size _
This error usually happens when you try to index a value that’s greater than the size of the array/list/DataFrame
/Series
. For example,
some_list = [2, 4, 6, 8]
some_list
has a length of 4. Trying some_list[6]
will error because index 6 is greater than the length of the array. Note that some_list[4]
will also cause an IndexError
because Python and pandas
uses zero indexing, which means that the first element has index 0, the second element has index 1, etc.; some_list[4]
would grab the fifth element, which is impossible when the list only has 4 elements.
ValueErrors
ValueError: Truth value of a Series is ambiguous
This error could occur when you apply Python logical operators (or
, and
, not
), which only operate on a single boolean values, to NumPy
arrays or Series
objects, which can contain multiple values. The fix is to use bitwise operators |
, &
, ~
, respectively, to allow for element-wise comparisons between values in arrays or Series
.
Alternatively, these errors could emerge due to overwriting Python keywords like bool
and sum
that may be used in the autograder tests, similar to what’s described here. You should follow a similar procedure of identifying the line of code erroring, checking if you’ve overwritten any Python keywords using Ctrl+F, and renaming those variables to something more informative before restarting your kernel and running the erroring tests again.
ValueError: Can only compare identically-labeled Series objects
As the message would suggest, this error occurs when comparing two Series
objects that have different lengths. You can double check the lengths of the Series
using len(series_name)
or series_name.size
.
ValueError: -1 is not in range
/ KeyError: -1
This error occurs when you try and index into a Series
or DataFrame
as you would a Python list. Unlike a list where passing an index of -1 gives the last element, pandas
interprets df[-1]
as an attempt to find the row corresponding to index -1 (that is, df.loc[-1]
). If your intention is to pick out the last row in df
, consider using integer-position based indexing by doing df.iloc[-1]
. In general, to avoid ambiguity in these cases, it is also good practice to write out both the row and column indices you want with df.iloc[-1, :]
.